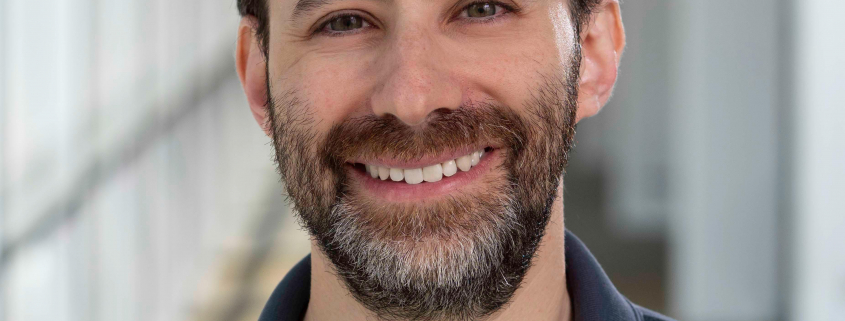
“A Theoretically-driven Approach to Measurement” Abstract: Item Response Theory (IRT) models are widely used in political science as a dimensional-reduction technique: they reduce variation in multiple variables to continuous variation along one or more latent dimensions. One-dimensional IRT models have a conceptual advantage in doing so in that the latent dimension they identify has intrinsic conceptual meaning, e.g., a student’s latent level of knowledge of the subject matter on an exam. That advantage dissipates when there is more than one latent dimension, however. When there are multiple latent dimensions, their meanings are typically assigned post-analysis, leading to potential bias in their assignment and a lack of reliability across data sources. We propose, detail, and validate on both simulated and real data a supervised approach employing Bayesian Item Response Theory on multiple latent dimensions and binary data. Our approach yields conceptually meaningful latent dimensions that are reliable across different data sources without additional exogenous assumptions. We focus on applications that employ extant survey data to make theoretically-meaningful measurements of concepts that are potentially different from what the survey was originally intended to measure. Our approach has applications to everything from conflict prediction to the assessment of psychological traits.